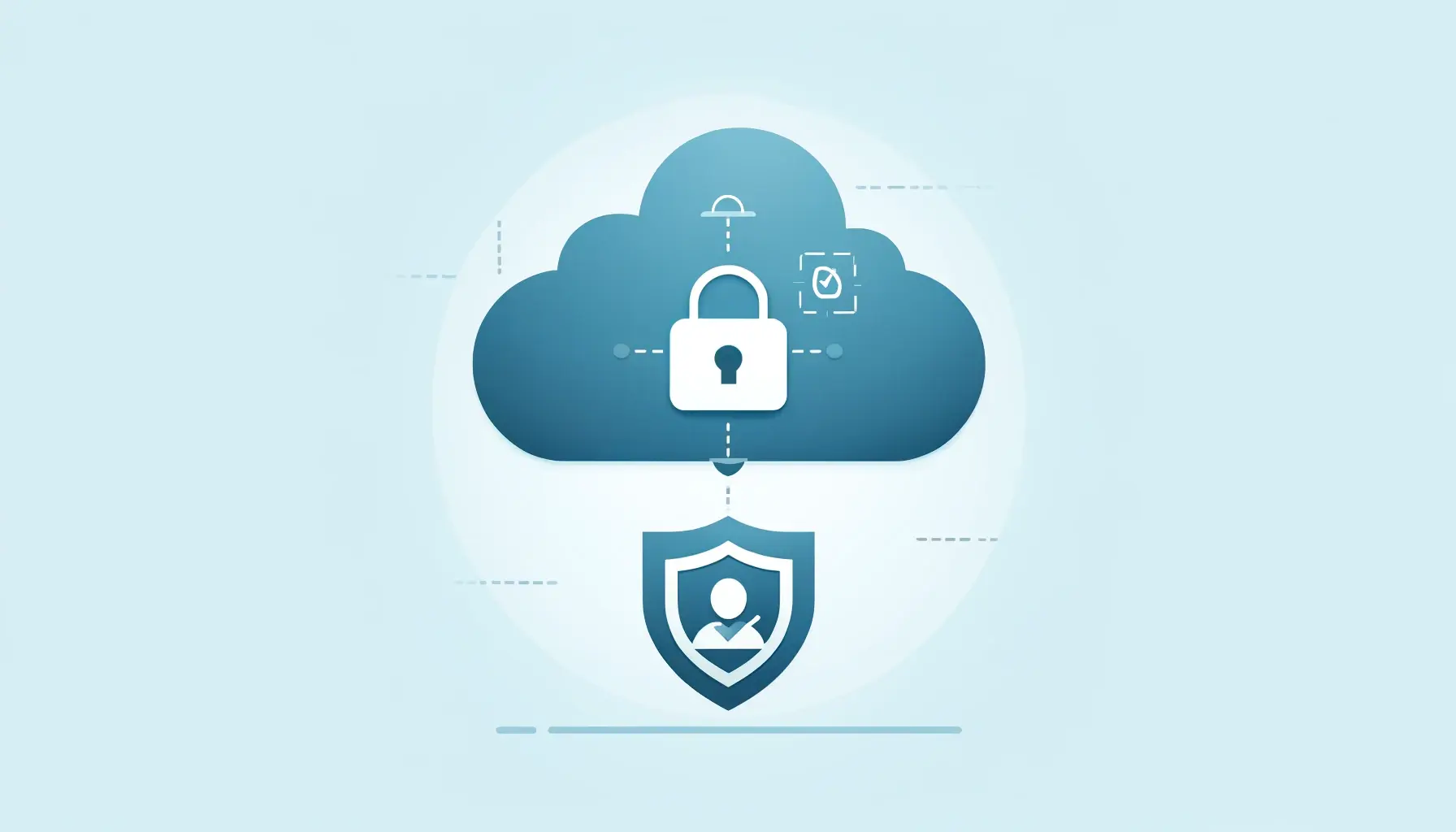
We're unlocking new business possibilities in the ascendancy of technologies like Large Language Models (LLMs) and generative AI. However, this innovation brings a surge in data security challenges, demanding a re-evaluation of data management and security postures.
Data isn't just a byproduct of this future; it's the driving force of technological advancements, especially AI. Developers need expansive data access to refine AI applications, posing significant challenges in safeguarding sensitive information and ensuring compliance.
Understanding AI's direction and nuances becomes indispensable in an AI-centric world. To ride this wave of innovation while safeguarding data assets, we must focus on prevailing AI trends, opportunities, and risks.
AI Trends, Opportunities, and Risks
- Refinement with Proprietary Data:
- Opportunity: Using internal data, businesses can sharpen their competitive edge, making products and services more responsive to customer needs.
- Risk: Proprietary data exposure to AI models for refinement can lead to breaches or inadvertent data disclosures.
- Real-time Data Interactions:
- Opportunity: AI frameworks interacting with data reservoirs in real-time elevate user experiences and immediacy in decision-making.
- Risk: Each interaction poses a vulnerability, challenging the security of each data access point.
- Enterprise-Specific LLM Development:
- Opportunity: Bespoke AI models offer insights and solutions for specific organizational goals.
- Risk: Custom development can result in AI "memorizing" sensitive information, posing security and ethical challenges.
Challenges Ahead: Data Access and Management
With data proliferation, aggregating data without compromising security becomes daunting. Developers need access to vast amounts of information, raising questions about secure and managed access.
Unraveling Complexities: Data Imprint Vulnerability & The Precipitation Risk
- Data Imprint Vulnerability: LLMs absorbing vast amounts of data can inadvertently retain and disclose sensitive information.
- The Precipitation Risk: Echoing the early cloud era, rapid AI deployment without robust security can lead to significant risks.
Crafting a Comprehensive Data Security Strategy in the LLM Era
Organizations must balance innovation with data security. Key strategy pillars include:
- Scrupulous Data Oversight: Rigorous mechanisms for assessing every data ingested by AI models.
- Proactive Risk Mitigation: Continuous monitoring during training to catch anomalies.
- Transparent AI Interactions: Maintaining visibility and real-time data interaction metrics.
- Eradicating Shadow Data: Regular scans identify unsanctioned data replicas and implement restrictive gateways.
Dasera: Data Security Posture Management For an AI-Dominant Era
As organizations embed AI into their core functionalities, Dasera, a data security posture management company (DSPM), emerges as a beacon of data assurance. With Dasera’s platform, organizations can proactively detect unauthorized access and potential data misuse, minimizing the impact of data breaches. As the world becomes increasingly data-driven, investing in security partnerships with innovative companies like Dasera is essential for safeguarding sensitive information, building customer trust, and ensuring a secure digital future.
We have reached the era where data and AI interplay shape our technological destiny, and the balance between potential and peril is critical. Companies like Dasera can help guide you through this complex journey. As we embrace an AI-driven future, ensuring data security isn't just a component; it's the foundation, aligning innovation with integrity. Let’s not make some of the same misconfiguration and data breach mistakes as we did with migration to the cloud; let’s proactively set the course of balancing security with innovation so we can all embrace and utilize the technological advancements of tomorrow.